Be Data Literate Part I: Why Aggregated Data Misleads, Misinforms, Misdirects
Add bookmark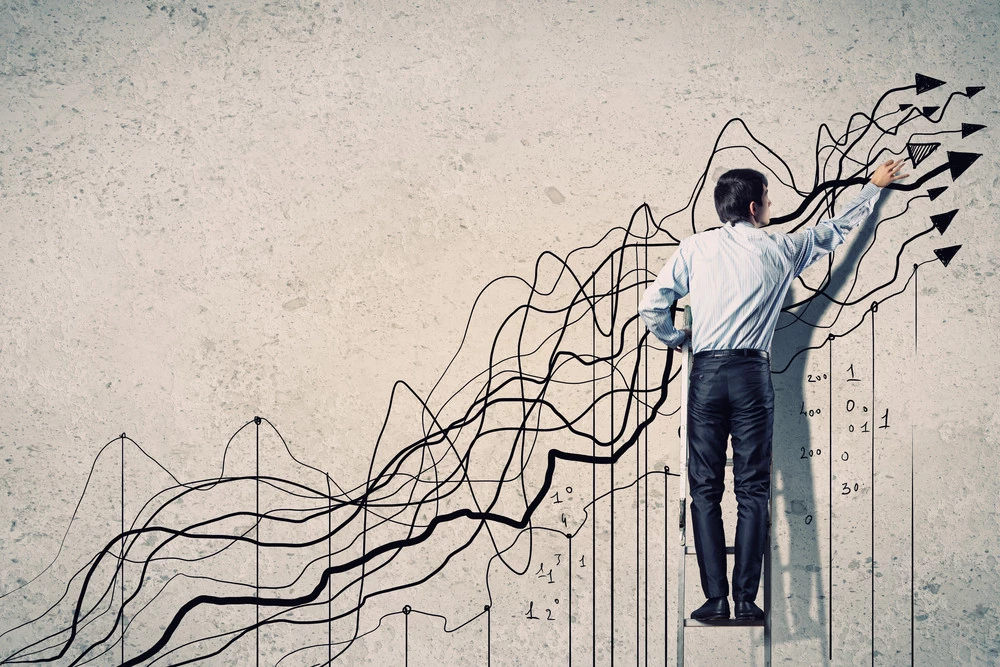
Editor's Note
This article discusses the need for analyzing data at the most meaningful level of aggregation (i.e., detail).
Parts I & II of this article attempts to illustrate why the diagnostic value of an overly aggregated metric/measurement is limited if not completely misleading.
Part III explains how to systematically disaggregate (i.e., continually refine data into relevant subgroups) when seeking to identify the root cause of a clearly identified problem.
Introduction
Many journalists aren't aware of it. Neither is corporate America. National magazines deny its existence. And public service organizations think it’s a process by which to extend the shelf life of milk.
It's called homogeneity. More precisely, homogeneity of data.
Simply put, homogeneity of data refers to whether or not the total data set from which measurements were computed conceals important differences between or among what statisticians call "rational subgroups or just plain subgroups."
This may sound, initially, complicated. It's not. Hang in there. It's worth the effort.
Preface To Homogeneity
To understand homogeneity, you must, first, understand what the term "aggregation" means. Nothing in this discussion should surprise you. Again, stay with it!
The degree of aggregation in data refers to the level of detail or refinement in data. A high level of aggregation conceals differences between and among subgroup categories. (In a few moments we will define what is meant by subgroups).
In order to reach the correct level of "homogeneity" with respect to your data you must organize the disaggregation task systematically, purposefully, with understanding, and with the objective of reaching the right conclusion with respect to a given metric and/or scorecard of metrics.
Memorize this. A high level of aggregation in a given data set conceals differences between and among subgroup categories. Should we say it again? We should. It will soon make sense –guaranteed!
But just memorize it for now! Got it? A high level of aggregation in your data conceals differences between and among important subgroup categories.
If you analyze a given metric at the wrong level of refinement or detail, chances are you'll fail to take the appropriate action.
What's a Subgroup?
Let's start with a very simple example. Suppose someone in your office decides to calculate the average weight of all office employees. Average weight is a measurement or metric.
The data collector makes no distinction between men and women. He/she simply gets everybody's weight, adds up the total weight and divides by the number of employees (total weight/total number of employees).
And the would-be data analyst proudly announces the average weight equals 160 pounds. Most definitely, you've already figured out what's wrong here.
In fancy (i.e., more technical) language, we would say the data set (men and women combined) is "overly-aggregated" with respect to the characteristic under study namely – weight of employees.
We have to subdivide the total data set into two relevant subgroups – men and women.
And that's what we do. And guess what happens? The average weight of all the women in your office equals 125 pounds and the average weight of all the men equals 159 pounds.
The two subgroups (men and women) revealed a significant difference in weight (i.e., 125 pounds vs. 159 pounds).
That would lead us to the conclusion the original data set (men and women combined) was non-homogeneous with respect to the measurement/characteristic under investigation, that is, weight.
Why? Because the original data set concealed significant differences in two relevant subgroups – men and women. The average in this case is totally misleading. It's what many statisticians call a meaningless statistic.
Next time you're presented with so-called evidence-based statistics–whether in the workplace or on cable news–ask yourself the following questions: is the statistic or measurement overly aggregated? Is the statistic or measurement concealing important differences between and among relevant subgroups?
We can't escape it. Everything from crime rates among illegal aliens to wage gap statistics must be put to the homogeneous data/overly aggregated data test.
In far too many cases, we're being presented with data that conceal significant differences among important subgroups.
A More Rigorous Definition of Homogeneity
Hopefully, you're now ready for a more rigorous definition of homogeneity. Read this definition very carefully. You should, given the above discussion, have no difficulty whatsoever in understanding what it says.
If a group of items, or set of data, can be sub-classified on the basis of a pertinent characteristic other than the one under investigation, into subgroups which yield significantly different values for the characteristic under investigation, then the group of items or total data is said to be non-homogeneous with respect to that characteristic.
The characteristic under investigation in the above example was weight of employees. The pertinent characteristic, other than the one under investigation, was gender (i.e., males and females).
By subdividing the total data set into male and female subgroups, we noted a significant difference in the weight of males versus females.
Again, this leads us to the conclusion the original data set (males plus females) was non-homogeneous with respect to the characteristic under investigation (weight).
Take-home message: When we deal with overly-aggregated data, we may be misguided in our conclusions because we inspect the data from an incorrect perspective.
The basic question is whether the result for the total group being studied (i.e., total data set) conceals important differences between and among relevant subgroups. If it does, chances are you'll jump to the wrong conclusion.
A Caveat
A group/data set may be homogeneous with respect to one characteristic or measurement, and at the same time, not be homogeneous with respect to another.
The set of people reading this article may very well be homogeneous with respect to I.Q. and educational attainment but not homogeneous with respect to weight.
Another Simple Example
Is your data overly-aggregated?
Assume it is! You can never go wrong with this mind set.
Although most people aren't aware of it, the key to finding the root cause of many problems is to “disaggregate” an aggregate number.
(In Part III we'll provide an example of the subdivide, subdivide, subdivide process to discover the root cause of a well-defined problem.)
So, what's the first step in the disaggregation process? Find relevant sub-aggregates or subgroups. This requires thinking and knowledge of the particular situation.
The percentage of delayed flights for a given airline is an “aggregate number.”' How would you subdivide "delayed flights" into relevant subgroups?
If delayed flights are subdivided into subgroups representing delayed flights at five airports, the resulting numbers represent "sub-aggregates" or "partitions." You, however, can call them subgroups. Your choice!
But from experience we've learned that when you call subgroups sub-aggregates or partitions, people think you're smarter and more data literate.
To repeat: The degree of aggregation in data refers to the level of detail or refinement in data. A high level of aggregation conceals differences between and among subgroup categories.
Most people realize “analysis of disaggregated data” may reveal important problems.
For example, if the number of delayed flights increased in the aggregate, the number of delayed flights may have still have decreased in four out of the five airports.
One airport may account for the overall, that is, aggregate increases in delayed flights because of, say, extremely bad weather conditions or other assignable causes.
This aggregate increase in delayed flights “conceals what’s really happening” and could prevent the correct remedial action.
Summary & Conclusions
We may be misguided in our conclusions if we inspect data from an incorrect perspective.
This is always possible, and only a careful consideration based on knowledge of the area or what's being investigated can minimize the danger of such a risk.
In Part II, we will provide several illuminating examples of how aggregated data causes decision-makers to jump to hasty – and in many cases downright wrong – conclusions.