Data Are Not Information – Raising The Analytics IQ of Managers/Executives
Add bookmark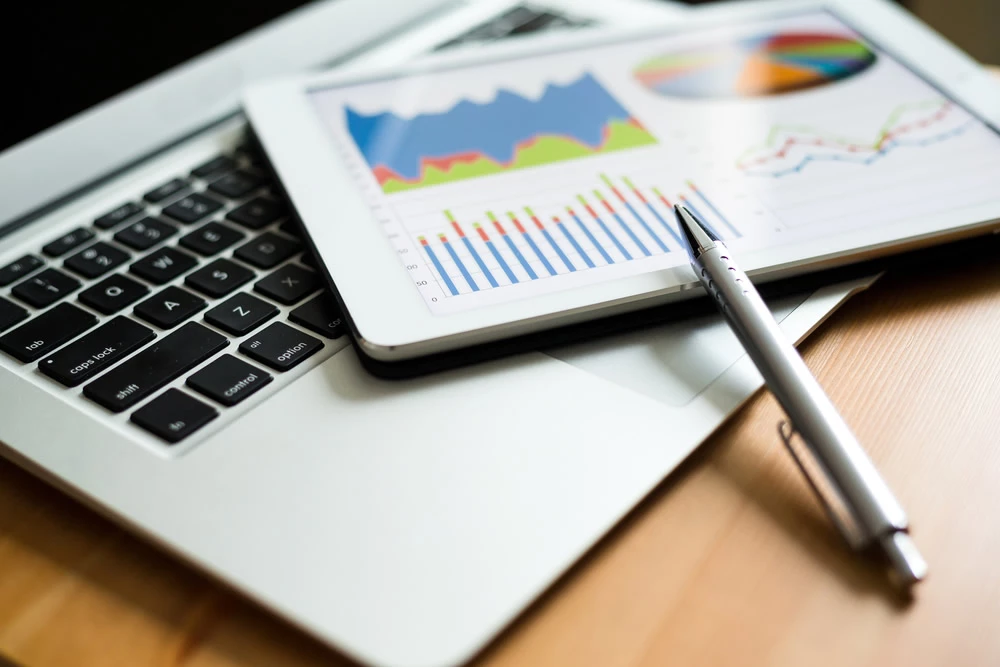
Editor's Note
Data-Smart. It's a hot topic these days–in books, in the business press and in management meetings.
It's not easy to forecast what progress will be made in the application of data analytics to management problem solving during the next decade.
It appears obvious, however, that within this period many new and not-so-new quantitative methodologies/analytics will be used to help managers better allocate resources, locate the root cause of reoccurring problems and improve predictions about future happenings.
Quick Digression: It should be noted–for those with an understanding of basic statistics–Artificial Intelligence (AI) is firmly rooted in multiple regression analysis. That's all we'll say about this for now. But will devote an entire article to explaining–in plain English–exactly what this means and why it's important.
Classifying Data Analytics Categories
Data analytics breaks down into three distinct categories–namely: (1) descriptive analytics; (2) prescriptive analytics and; (3) predictive analytics.
It should be mentioned–indeed, emphasized–the term "analytics" is relatively new. Years ago, the three above terms were called descriptive statistics, prescriptive statistics and predictive statistics. Message: "Old wine in new bottles."
Further, what matters in today's management world is whether data analytics methodologies, old or new, are applicable to solving a given problem.
For example, the movie Hidden Figures illustrated how Newtonian physics & Euclidean geometry were applicable to the early beginnings of the space program.
What is relevant is the imagination and the skill of whoever applies a given methodology or technique, rather than the sophistication or newness of methodology.
This is quite obvious. But it bears repeating in today's new world of fast forgetting and slow learning.
Data, Information, Knowledge & Wisdom
Before we begin, let's make some distinctions. There are vast differences between data, information and knowledge.
Data collected with no specific purpose in mind, tends to become unwieldy and cumbersome. It just sits there and gathers cobwebs. In short, it's useless.
So, the next step is to process/analyze the data, that is, convert it into meaningful information. This can mean a lot of things.
To the trained statistician (a.k.a. data scientist) it means organizing and making sense of the data. An often used phrase by professional statisticians drives this point home: “Make your data talk.”
Data scientists trained in the rigors of statistical analysis have their own “black bag” of specialized instruments for converting data into information.
“A Bell's not a Bell 'til you Ring it, A Song's not a Song 'til you Sing it”
Extracting useful information from the data doesn't always lead to meaningful action, that is, it doesn't mean it will be applied to specific work and performance.
If the information is put to work or into practice, it is said to become KNOWLEDGE. And that only comes with a human being, his/her brain or the skill of his hands.
If the individual does not have the training, learned skills, incentive or willingness to put valuable information to work, it will not become knowledge. And its knowledge that creates corporate wealth.
This is not a new insight for training professionals. To slightly paraphrase Peter F. Drucker: “unless managers can immediately put into action the things they have learned, the instructional programs will not 'take.' It will remain 'information' and never become 'knowledge'…'”
Take-home message: Data can be effectively arranged and converted to meaning without adding value or creating wealth for the organization. Unless the information is turned into knowledge, that is, directed toward specific work and performance, much of the effort is wasted.
Many organizations spend far too much on information acquisition, leading to comments like, “We are drowning in information and starved for “knowledge.”
In short, there are vast differences between data, information, and knowledge.
Now try to explain this to the next person that's willing listen to you. Don't be a bore. But remember the best way to learn a subject is to teach it!
Now… A Short Commentary On Wisdom
In 1973 Princeton's John Turkey wrote a book entitled Exploratory Data Analysis, which detailed "mining data" methodologies for collecting and classifying information, evaluating its quality, looking for hidden relationships in mission-critical data and much more.
Take for example a major retailing company who–selling primarily through catalogs with over 4 million customers–decided to invite a leading customer relationship management organization to mine its data and help it discover customer groupings.
Instead of coming up with the usual five or six segments, or 50 niches classified by demographics, usage rates, and the like, the data mining experts identified 8,000 market cells.
Translated, they found 1,000 current customers of the catalog company had recently purchased a blue shirt and a new tie. Oversimplifying, these buyers would probably be more interested in buying, say, a navy blue blazer than the average customer of the catalog company.
The point? It may pay for the catalog company to promote just to these 1,000 customers a special customer discount on a navy blue blazer. If the company is correct, this specific niche or market cell's response rate to the offer could be 30% or 40% higher than the typical response ratio if promoted fast and successfully.
Our point? Once again we repeat there are vast differences between data, information, knowledge, and wisdom.
Unless data are processed into information, which is turned into knowledge, which (in this case) becomes market wisdom, much of it is wasted.
Descriptive Analytics
The first step in accomplishing the data into useful information task comes under the category known as Descriptive Analytics (formerly known as descriptive statistics).
Managers are not philosophers. They want more than just numbers. They want numbers organized so as to convey information. Information they can put into immediate practice to achieve a desired result.
It must be mentioned–indeed, emphasized– managers, executives and other knowledge workers can learn relatively simple statistical concepts and procedures to:
- Tackle basic measurement and operational definition problems
- Avoid being foold by far-fetched estimates
- Immediately spot cheating charts
- Use the right kind of average that correctly summarizes data sets
- Compute a measure of variation/dispersion to determine if a given average provides the least distorted one-figure summary of the data
- Make proper (as opposed to improper) comparisons of information
- And much more!
Descriptive statistics, after all is said and done, embodies a set of easy-to-use tools for converting data into useful, reliable statistical information. In brief, executives/managers and others need to learn in a very practical way the uses & abuses descriptive statistics.
Our point? Today, the teaching of descriptive analytics is the first step in what is now called “raising the analytics IQ of knowledge (i.e., professional, managerial, and technical) workers.”
Unfortunately many basic statistics courses in universities and colleges across the nation do not go beyond descriptive statistics. Worse, it seems much of the content taught in basic statistics boils down to "techniques looking for problems."
That said, many top-tier schools still require a one year statistics course that covers the basic foundational knowledges necessary to understanding and using prescriptive and predictive analytics.
Prescriptive Analytics
Today's manager needs more than descriptive analytics. They need to understand what W. Edwards Deming and many others have preached for nearly 60 years–how to use basic statistical analysis as a tool (e.g., finding the root cause of a given problem by sequential disaggregation of data and, then, prescribing a remedy.
We strongly suggest you read the disaggregation article before proceeding. You will, we truly believe, have a far better understanding of what a prescriptive statistical methodology is and why it's such a powerful managerial tool.
Predictive Analytics
The use of predictive analytics for making better, say, sales forecasts, productivity improvement estimates, and a whole host of other problems that require making reliable predictions… and then measuring predicted results versus actual results is now an extremely important subject given the many statistical computer programs for doing this.
Like all statistical methods, predictive analytics (i.e., time series/regression/correlation analyses)) should be used only after careful thought shows it's an appropriate approach to a given problem.
Much of the underlying mathematical/statistical theory of the body of tools that comprise predictive analytics is simple and generalizes easily; yet the application of these methods to data analysis leads to numerical tables and graphs which give the impression of being extremely scientific.
But this is not always the case if the analysis is done by people not thoroughly trained in statistical analysis.
Today's manager must learn how to interpret the results of plug-in and solve equations, and understand things like the difference between cross-sectional and times-series data-(i.e. data collected at a point in time versus data collected over a long period of time). Big difference in interpreting results.
However, many data into information conversion problems require very advanced statistical techniques. For these type problems, professional data analysts should/must be consulted.
A Simple Example of Predictive Analytics With A Link To An Example You'll Never Forget
On Sunday, February 16, 2012, The New York Times Magazine published an article: How Companies Learn Your Secrets, by Charles Duhigg.
It’s a great article on the use of predictive analytics, especially for CLOs, CEOs, CFOs, marketing executives, marketing researchers and data scientists.
Training professionals charged with the responsibility of raising the analytics IQ of their organization will welcome the insights provided by Duhigg. We recommend you take the time to read his article.
In essence, Duhigg explains how Target (the giant retailer) applies predictive analytics to identify “ information” in their CRM system to predict whether a female shopper is expecting a baby.
A baby? That's right. A baby. Once this is/was established, Target is/was enabled to reach potential customers (long before rivals) for their diaper home delivery service.
Again, we strongly suggest you read the New York Times article. By so doing, coupled with the above-mentioned article on disaggregating data to determine the root cause of a problem, you will have greatly increased your understanding of today's new analytics. (Please let us know if we are correct in our assessment).