Be Data Literate Part II: Useful Examples of the Interpretation Dangers Of Overly-Aggregated Data
Add bookmark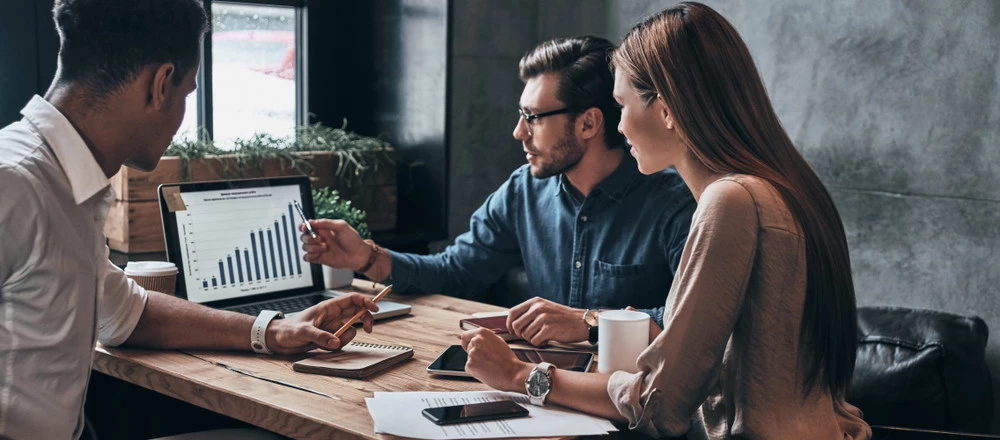
Editor's Note
Part I of this article explained why you may be misguided with respect to so-called data-driven conclusions because you're inspecting/evaluating the data from an incorrect perspective.
This is always possible, and only a careful consideration of how to subdivide a given data set into the appropriate subgroups can minimize the danger of such a risk.
In Part II, we provide readers with several sub-grouping examples (two taken from today's front-line news) that provide you with a deeper understanding of the dangers of statistical ignorance with respect to failure to analyze presented metrics or data at the appropriate level of aggregation.
Introduction
Many years ago, one of our editors read a news story citing the significant difference between the points scored by men and women on mathematical aptitude tests in several university-administered examinations (not related to SAT scores).
Re-creating this long-ago story, the average for men was reported at 560 points and the average for women was 521.
A difference of this magnitude, had all sorts of implications (or so went the rhetoric) concerning the teaching of quantitatively oriented courses.
Radically different male versus female performances, however, had not been observed in these courses, and the data was inspected further.
Examination of the scores for men and women within various professional schools within the universities supplied the rather simple explanation.
Mathematical aptitude scores in the engineering schools at the universities studied were high for both sexes; but (at the time) there were very few women enrolled in the engineering schools.
The schools of nursing, on the other hand, were almost exclusively composed of women, and most students in the nursing schools recorded significantly lower scores in the mathematical aptitude examinations given.
The appropriate data are given in Table I and seems to indicate males and females within a given professional school had very similar averages.
Table I: Average Mathematical Aptitude Scores of Men And Woman By School Within Several Universities
Average Score | ||
School | Men | Women |
Engineering | 610 | 608 |
Liberal Arts | 514 | 517 |
Nursing | 467 | 473 |
Others | 526 | 522 |
Average | 560 | 521 |
What superficially appears to be a difference associated with gender, became, on closer examination, a difference associated with professional school.
Within a given professional school men and women are "homogeneous" with respect to mathematical aptitude.
Behind the Newspaper Headlines
Heather Mac Donald, in her important and timely book entitled The War On Cops, provides us with a clear-eyed analysis of real-world policing and the importance of basic measurement and definition problems.
Ms. Mac Donald separates truth from fiction through informed street-level reporting coupled with a solid background in putting into practice timeless statistical procedures.
In her book, she illustrates how a meaningless statistic goes unnoted and causes much civil unrest. For example, it was reported that "Black men accounted for 40% of the 60 'unarmed deaths' {by police officers} to date."
Step-by-step, Ms. Mac Donald peels away the onion. For starters, the 24 unarmed Black men killed by the police constituted a surprisingly small fraction of the 585 victims of police shootings. Further, most of the 585 victims were White or Hispanic (and most were armed).
Relevant to our discussion on homogeneity, Ms. Mac Donald examines the "unarmed victim" profile in great detail.
Unarmed, she notes, might be correct; but this label fails to convey the number of cases (five) that involved Black victims resisting arrest and attempting to grab the police officer's revolver.
What if we can subdivide Black victims into two subgroups – namely, resisting arrest and not resisting arrest. Would we get a different answer? Ms. Mac Donald's disaggregation of the data provided much illumination about a much publicized happening.
This is, unquestionably, an oversimplification. But it does make the point. Before rushing to erroneous conclusions, relevant data sets must be disaggregated.
Measuring Patient Outcomes: The Potential Effects of Lurking Subgroups
The above examples illustrate the effect of subgroups that can invalidate a presented measurement. We now continue with a more practical illustration taken from today's news headlines.
In an attempt to help people make informed decisions about health care options, the government releases data about patient outcomes for a large number of hospitals.
Further, hospitals, in many instances, are financially rewarded on the basis of "patient outcomes." Measurements define what we mean by performance. Defining what is meant by patient outcomes and determining how to measure it is an essential task.
Many learned articles have been published about the benefits and dangers of this relatively new happening. To think through the appropriate measurement(s) for patient outcomes is in itself a policy decision and therefore highly risky.
Measurement directs effort and vision; Measurement determines where efforts should be spent.
Improving patient outcome measurements has become the raison d'être for an increasing number of hospitals in, large part, because of the associated financial rewards accruing to those with improved scores.
David S. Moore & George P. McCabe's outstanding applied data analysis textbook, Introduction to the Practice of Statistics (2007) provides a hypothetical example of what can go wrong with patient outcome measurements – and potentially produce an unexpected/unwanted result:
"You are interested in comparing Hospital A and Hospital B, which serve your community. Here are the data on the survival of patients after a specific kind of surgery in these two hospitals.
All patients undergoing surgery in a recent time period are included; 'survived' means that the patient lived at least six weeks following surgery.
Table 2
Outcome | Hospital A | Hospital B |
Died | 63 | 16 |
Survived | 2037 | 784 |
Total | 2100 | 800 |
The evidence seems clear: Hospital A loses 3% (63/2100) of its surgery patients, whereas Hospital B loses only 2% (16/800)."
You should choose Hospital B (2% mortality) if you need surgery. At least, that's what the "aggregated" data reveals."
But you now know a high level of aggregation conceals differences between and among subgroup categories. Unfortunately, the very patients the government wants to protect are unaware of this statistical truism.
Sub-classifying by Patient Condition: Disaggregating Patient Outcome Data
Let's assume, later in the government report you find data on the outcome of surgery broken down by the condition of the patient before the operation.
"Patients are classified as in either 'poor' or 'good' condition. Table 3 shows more detailed data.
Table 3
Good Condition | Bad Condition | ||||
Hospital A | Hospital B | Hospital A | Hospital B | ||
Died | 6 | 8 | Died | 57 | 8 |
Survived | 594 | 592 | Survived | 1443 | 192 |
Total | 600 | 600 | Total | 1500 | 200 |
What does table 3 tell you? For starters, it says:
- Hospital A beats Hospital B in patients in good condition: only 1% (6/600) died in Hospital A, compared with 1.3% (8/600) in Hospital B.
- Hospital A wins again for patients in poor condition, losing 3.8% (57/1500) to Hospital B's 4% (8/200).
Conclusion: Hospital A is safer for both patients in good condition and patients in poor condition. If you're facing surgery, you should choose Hospital A." But you wouldn't know to select Hospital A if presented with the aggregated metric.
Let's be harshly realistic. If you're a hospital administrator seeking the full benefit of government rewards for better patient outcomes, you may be inclined to reject "patients in poor condition" in order to improve your aggregate patient outcome metric.
Critics of "better pay for better patient outcomes" have been quite vocal about this unintended consequence and claim some hospitals are, indeed, sending poor condition patients elsewhere in order to maintain a desirable patient outcome result in order to continually receive financial rewards.
Another Possible Subgrouping For Studying Patient Outcomes
Patient outcomes, among many other factors, could very well be physician/surgeon-specific. It would be interesting to see a breakdown of outcomes by, say, the surgeons performing a specific type of procedure.
There's an old statistical joke relating to this. Probability is measured by the relative frequency of an event in the long run.
It doesn't mean what the hypothetical physician thought it meant, when, she said: "I'm sorry to say you have a very serious disease… As a matter of fact, 9 out of every 10 people who have this disease die of it…
… But you are very lucky you came to me… You see, the last nine people I examined/treated who had the disease, died."
Check out Part III where we present a simple (but not simplistic) statistical approach for "disaggregating" data to identify what statisticians sometimes call "an assignable cause.”